Machine learning models are increasingly being utilized in working memory training, offering new possibilities for personalized and adaptive interventions. This essay explores the applications of machine learning in working memory training, highlighting its potential benefits and current limitations.
Predicting Training Outcomes
One of the primary applications of machine learning in working memory training is predicting individual outcomes. Researchers have developed models that can forecast whether a participant is likely to benefit from a specific training protocol. For instance, Vladisauskas et al. (2022) trained a support vector classifier using baseline individual differences as features to predict improvements in executive functions among 6-year-olds. The model achieved an average accuracy of 0.67 and an AUC of 0.707, demonstrating the potential of machine learning in identifying individuals who are more likely to benefit from cognitive training interventions.
Personalizing Training Protocols
Machine learning algorithms can help tailor working memory training programs to individual needs. By analyzing patterns in performance data, these models can adapt the difficulty and content of training tasks in real-time. Seitz et al. (2020) examined how Bayesian filtering approaches, such as hidden Markov models and Kalman filters, and deep-learning approaches, like long short-term memory (LSTM) models, can be used to estimate user skill level and predict appropriate task challenges. Their results showed that hidden Markov models were most accurate in predicting participants’ performances as a function of provided challenges, thus placing participants at appropriate future challenges.
Tracking Skill Development
Another promising application of machine learning in working memory training is tracking participant skills throughout the training process. Zhang et al. (2020) investigated the effects of working memory span training on working memory maintenance using event-related potential (ERP) evidence. Their study demonstrated that machine learning models could be used to analyze ERP data and track changes in working memory processes over the course of training.
Identifying Influential Factors
Machine learning models can help identify the factors that contribute most significantly to training outcomes. Katz et al. (2023) applied machine learning algorithms to predict individuals’ training patterns based on several individual difference variables, including pre-existing cognitive abilities, personality characteristics, and motivational factors[4]. They found that openness and pre-existing working memory capacity were the two most important factors in distinguishing between high and low performers.
Challenges and Future Directions
While the application of machine learning in working memory training shows promise, several challenges remain. The limited availability of large, high-quality datasets is a significant obstacle to developing more robust and generalizable models. Additionally, the complexity of some machine learning models can make it difficult to interpret their predictions, potentially limiting their practical application in clinical settings.
Future research should focus on developing larger, more diverse datasets to train and validate machine learning models. There is also a need for more studies directly comparing the effectiveness of machine learning-guided interventions to traditional approaches. As the field progresses, it will be crucial to address ethical considerations surrounding the use of AI in cognitive training, ensuring that these tools are used responsibly and equitably.
In conclusion, machine learning models offer exciting possibilities for enhancing working memory training. By enabling personalized interventions, predicting outcomes, and providing insights into the factors influencing training success, these tools have the potential to significantly improve the effectiveness of cognitive training programs. However, continued research and development are necessary to fully realize the benefits of machine learning in this field.
References
Here’s the list of references rewritten in APA 7th edition style:
References:
Katz, B., Buschkuehl, M., Shah, P., Jaeggi, S. M., & Jonides, J. (2023). Unicorn, hare, or tortoise? Using machine learning to predict working memory training performance. Journal of Cognition, 6(1), 53. https://doi.org/10.5334/joc.263
Seitz, A. R., Pahor, A., Jaeggi, S. M., & Shah, P. (2020). Application of machine learning models for tracking participant skill in cognitive training studies. Frontiers in Psychology, 11, 1532. https://doi.org/10.3389/fpsyg.2020.01532
Vladisauskas, M., Belloli, L. M. L., Sánchez-Peña, R. S., Lipina, S. J., & Goldin, A. P. (2022). A machine learning approach to personalize computerized cognitive training interventions. Frontiers in Psychology, 13, 758262. https://doi.org/10.3389/fpsyg.2022.758262
Zhang, Q., Li, Y., Zhao, W., Chen, X., Li, X., Du, B., Deng, X., Ji, F., Wang, C., Xiang, Y., Dong, Q., Jaeggi, S. M., Chen, C., Song, Y., & Li, J. (2020). ERP evidence for the effect of working memory span training on working memory maintenance: A randomized controlled trial. Neurobiology of Learning and Memory, 167, 107129. https://doi.org/10.1016/j.nlm.2019.107129
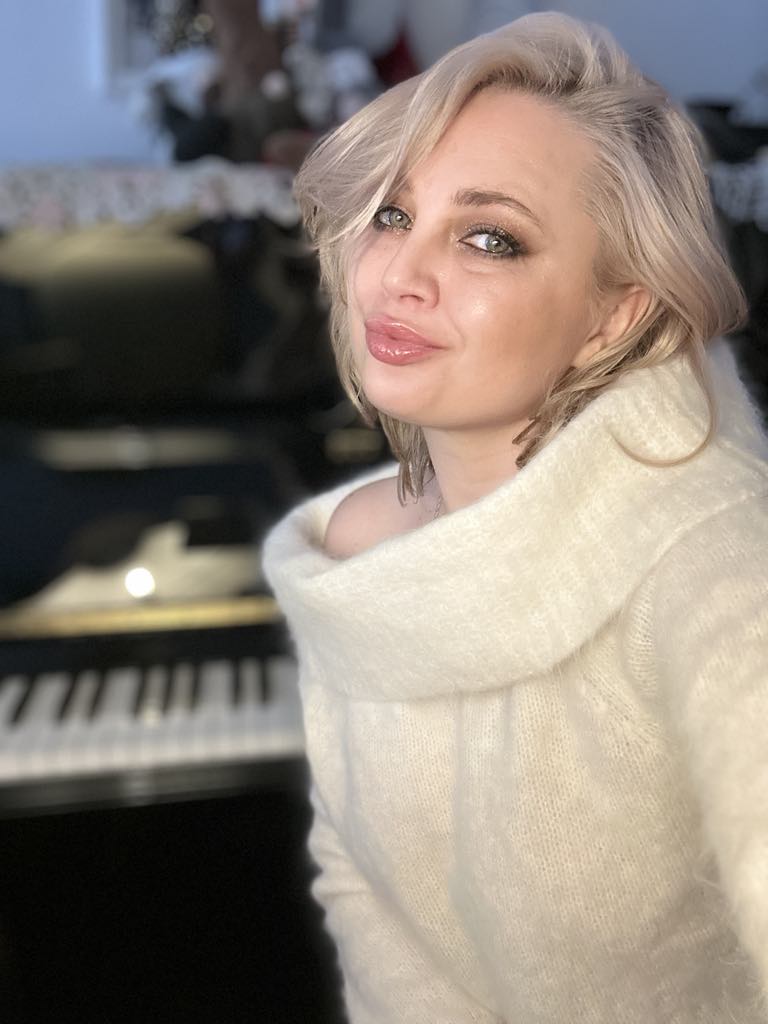
As a research scientist in cognitive neuroscience and psychology, I write a blog exploring computational modeling and gamified working memory training. I share insights from my research on how these approaches impact learning and cognition in both typical and clinical populations, with a focus on cognitive rehabilitation for brain injuries, neurodegenerative, and neurodevelopmental conditions. My blog also covers cognitive, emotional, and behavioral assessment, the influence of biopsychosocial factors, and the application of machine learning in neuropsychological interventions. By translating complex science into accessible content, I aim to inform professionals and the public about brain health and cognitive science.
Dorota Styk